algorithms.statistics.models.family.family¶
Module: algorithms.statistics.models.family.family
¶
Inheritance diagram for nipy.algorithms.statistics.models.family.family
:
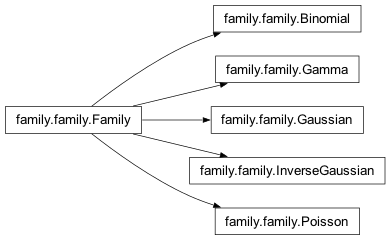
Classes¶
Binomial
¶
- class nipy.algorithms.statistics.models.family.family.Binomial(link=<nipy.algorithms.statistics.models.family.links.Logit object>, n=1)¶
Bases:
Family
Binomial exponential family.
- INPUTS:
link – a Link instance n – number of trials for Binomial
- __init__(link=<nipy.algorithms.statistics.models.family.links.Logit object>, n=1)¶
- deviance(Y, mu, scale=1.0)¶
Deviance of (Y,mu) pair. Deviance is usually defined as the difference
DEV = (SUM_i -2 log Likelihood(Y_i,mu_i) + 2 log Likelihood(mu_i,mu_i)) / scale
- INPUTS:
Y – response variable mu – mean parameter scale – optional scale in denominator of deviance
- OUTPUTS: dev
dev – DEV, as described above
- devresid(Y, mu)¶
Binomial deviance residual
- INPUTS:
Y – response variable mu – mean parameter
- OUTPUTS: resid
resid – deviance residuals
- fitted(eta)¶
Fitted values based on linear predictors eta.
- INPUTS:
- eta – values of linear predictors, say,
X beta in a generalized linear model.
- OUTPUTS: mu
mu – link.inverse(eta), mean parameter based on eta
- property link¶
- links = [<nipy.algorithms.statistics.models.family.links.Logit object>, <nipy.algorithms.statistics.models.family.links.CDFLink object>, <nipy.algorithms.statistics.models.family.links.CDFLink object>, <nipy.algorithms.statistics.models.family.links.Log object>, <nipy.algorithms.statistics.models.family.links.CLogLog object>]¶
- predict(mu)¶
Linear predictors based on given mu values.
- INPUTS:
mu – mean parameter of one-parameter exponential family
- OUTPUTS: eta
- eta – link(mu), linear predictors, based on
mean parameters mu
- tol = 1e-05¶
- valid = [-inf, inf]¶
- variance = <nipy.algorithms.statistics.models.family.varfuncs.Binomial object>¶
- weights(mu)¶
Weights for IRLS step.
w = 1 / (link’(mu)**2 * variance(mu))
- INPUTS:
mu – mean parameter in exponential family
- OUTPUTS:
w – weights used in WLS step of GLM/GAM fit
Family
¶
- class nipy.algorithms.statistics.models.family.family.Family(link, variance)¶
Bases:
object
A class to model one-parameter exponential families.
- INPUTS:
link – a Link instance variance – a variance function (models means as a function
of mean)
- __init__(link, variance)¶
- deviance(Y, mu, scale=1.0)¶
Deviance of (Y,mu) pair. Deviance is usually defined as the difference
DEV = (SUM_i -2 log Likelihood(Y_i,mu_i) + 2 log Likelihood(mu_i,mu_i)) / scale
- INPUTS:
Y – response variable mu – mean parameter scale – optional scale in denominator of deviance
- OUTPUTS: dev
dev – DEV, as described above
- devresid(Y, mu)¶
The deviance residuals, defined as the residuals in the deviance.
Without knowing the link, they default to Pearson residuals
resid_P = (Y - mu) * sqrt(weight(mu))
- INPUTS:
Y – response variable mu – mean parameter
- OUTPUTS: resid
resid – deviance residuals
- fitted(eta)¶
Fitted values based on linear predictors eta.
- INPUTS:
- eta – values of linear predictors, say,
X beta in a generalized linear model.
- OUTPUTS: mu
mu – link.inverse(eta), mean parameter based on eta
- property link¶
- links = []¶
- predict(mu)¶
Linear predictors based on given mu values.
- INPUTS:
mu – mean parameter of one-parameter exponential family
- OUTPUTS: eta
- eta – link(mu), linear predictors, based on
mean parameters mu
- tol = 1e-05¶
- valid = [-inf, inf]¶
- weights(mu)¶
Weights for IRLS step.
w = 1 / (link’(mu)**2 * variance(mu))
- INPUTS:
mu – mean parameter in exponential family
- OUTPUTS:
w – weights used in WLS step of GLM/GAM fit
Gamma
¶
- class nipy.algorithms.statistics.models.family.family.Gamma(link=<nipy.algorithms.statistics.models.family.links.Power object>)¶
Bases:
Family
Gamma exponential family.
- INPUTS:
link – a Link instance
- BUGS:
no deviance residuals?
- __init__(link=<nipy.algorithms.statistics.models.family.links.Power object>)¶
- deviance(Y, mu, scale=1.0)¶
Deviance of (Y,mu) pair. Deviance is usually defined as the difference
DEV = (SUM_i -2 log Likelihood(Y_i,mu_i) + 2 log Likelihood(mu_i,mu_i)) / scale
- INPUTS:
Y – response variable mu – mean parameter scale – optional scale in denominator of deviance
- OUTPUTS: dev
dev – DEV, as described above
- devresid(Y, mu)¶
The deviance residuals, defined as the residuals in the deviance.
Without knowing the link, they default to Pearson residuals
resid_P = (Y - mu) * sqrt(weight(mu))
- INPUTS:
Y – response variable mu – mean parameter
- OUTPUTS: resid
resid – deviance residuals
- fitted(eta)¶
Fitted values based on linear predictors eta.
- INPUTS:
- eta – values of linear predictors, say,
X beta in a generalized linear model.
- OUTPUTS: mu
mu – link.inverse(eta), mean parameter based on eta
- property link¶
- links = [<nipy.algorithms.statistics.models.family.links.Log object>, <nipy.algorithms.statistics.models.family.links.Power object>, <nipy.algorithms.statistics.models.family.links.Power object>]¶
- predict(mu)¶
Linear predictors based on given mu values.
- INPUTS:
mu – mean parameter of one-parameter exponential family
- OUTPUTS: eta
- eta – link(mu), linear predictors, based on
mean parameters mu
- tol = 1e-05¶
- valid = [-inf, inf]¶
- variance = <nipy.algorithms.statistics.models.family.varfuncs.Power object>¶
- weights(mu)¶
Weights for IRLS step.
w = 1 / (link’(mu)**2 * variance(mu))
- INPUTS:
mu – mean parameter in exponential family
- OUTPUTS:
w – weights used in WLS step of GLM/GAM fit
Gaussian
¶
- class nipy.algorithms.statistics.models.family.family.Gaussian(link=<nipy.algorithms.statistics.models.family.links.Power object>)¶
Bases:
Family
Gaussian exponential family.
- INPUTS:
link – a Link instance
- __init__(link=<nipy.algorithms.statistics.models.family.links.Power object>)¶
- deviance(Y, mu, scale=1.0)¶
Deviance of (Y,mu) pair. Deviance is usually defined as the difference
DEV = (SUM_i -2 log Likelihood(Y_i,mu_i) + 2 log Likelihood(mu_i,mu_i)) / scale
- INPUTS:
Y – response variable mu – mean parameter scale – optional scale in denominator of deviance
- OUTPUTS: dev
dev – DEV, as described above
- devresid(Y, mu, scale=1.0)¶
Gaussian deviance residual
- INPUTS:
Y – response variable mu – mean parameter scale – optional scale in denominator (after taking sqrt)
- OUTPUTS: resid
resid – deviance residuals
- fitted(eta)¶
Fitted values based on linear predictors eta.
- INPUTS:
- eta – values of linear predictors, say,
X beta in a generalized linear model.
- OUTPUTS: mu
mu – link.inverse(eta), mean parameter based on eta
- property link¶
- links = [<nipy.algorithms.statistics.models.family.links.Log object>, <nipy.algorithms.statistics.models.family.links.Power object>, <nipy.algorithms.statistics.models.family.links.Power object>]¶
- predict(mu)¶
Linear predictors based on given mu values.
- INPUTS:
mu – mean parameter of one-parameter exponential family
- OUTPUTS: eta
- eta – link(mu), linear predictors, based on
mean parameters mu
- tol = 1e-05¶
- valid = [-inf, inf]¶
- variance = <nipy.algorithms.statistics.models.family.varfuncs.VarianceFunction object>¶
- weights(mu)¶
Weights for IRLS step.
w = 1 / (link’(mu)**2 * variance(mu))
- INPUTS:
mu – mean parameter in exponential family
- OUTPUTS:
w – weights used in WLS step of GLM/GAM fit
InverseGaussian
¶
- class nipy.algorithms.statistics.models.family.family.InverseGaussian(link=<nipy.algorithms.statistics.models.family.links.Power object>)¶
Bases:
Family
InverseGaussian exponential family.
- INPUTS:
link – a Link instance n – number of trials for Binomial
- __init__(link=<nipy.algorithms.statistics.models.family.links.Power object>)¶
- deviance(Y, mu, scale=1.0)¶
Deviance of (Y,mu) pair. Deviance is usually defined as the difference
DEV = (SUM_i -2 log Likelihood(Y_i,mu_i) + 2 log Likelihood(mu_i,mu_i)) / scale
- INPUTS:
Y – response variable mu – mean parameter scale – optional scale in denominator of deviance
- OUTPUTS: dev
dev – DEV, as described above
- devresid(Y, mu)¶
The deviance residuals, defined as the residuals in the deviance.
Without knowing the link, they default to Pearson residuals
resid_P = (Y - mu) * sqrt(weight(mu))
- INPUTS:
Y – response variable mu – mean parameter
- OUTPUTS: resid
resid – deviance residuals
- fitted(eta)¶
Fitted values based on linear predictors eta.
- INPUTS:
- eta – values of linear predictors, say,
X beta in a generalized linear model.
- OUTPUTS: mu
mu – link.inverse(eta), mean parameter based on eta
- property link¶
- links = [<nipy.algorithms.statistics.models.family.links.Power object>, <nipy.algorithms.statistics.models.family.links.Power object>, <nipy.algorithms.statistics.models.family.links.Power object>, <nipy.algorithms.statistics.models.family.links.Log object>]¶
- predict(mu)¶
Linear predictors based on given mu values.
- INPUTS:
mu – mean parameter of one-parameter exponential family
- OUTPUTS: eta
- eta – link(mu), linear predictors, based on
mean parameters mu
- tol = 1e-05¶
- valid = [-inf, inf]¶
- variance = <nipy.algorithms.statistics.models.family.varfuncs.Power object>¶
- weights(mu)¶
Weights for IRLS step.
w = 1 / (link’(mu)**2 * variance(mu))
- INPUTS:
mu – mean parameter in exponential family
- OUTPUTS:
w – weights used in WLS step of GLM/GAM fit
Poisson
¶
- class nipy.algorithms.statistics.models.family.family.Poisson(link=<nipy.algorithms.statistics.models.family.links.Log object>)¶
Bases:
Family
Poisson exponential family.
- INPUTS:
link – a Link instance
- __init__(link=<nipy.algorithms.statistics.models.family.links.Log object>)¶
- deviance(Y, mu, scale=1.0)¶
Deviance of (Y,mu) pair. Deviance is usually defined as the difference
DEV = (SUM_i -2 log Likelihood(Y_i,mu_i) + 2 log Likelihood(mu_i,mu_i)) / scale
- INPUTS:
Y – response variable mu – mean parameter scale – optional scale in denominator of deviance
- OUTPUTS: dev
dev – DEV, as described above
- devresid(Y, mu)¶
Poisson deviance residual
- INPUTS:
Y – response variable mu – mean parameter
- OUTPUTS: resid
resid – deviance residuals
- fitted(eta)¶
Fitted values based on linear predictors eta.
- INPUTS:
- eta – values of linear predictors, say,
X beta in a generalized linear model.
- OUTPUTS: mu
mu – link.inverse(eta), mean parameter based on eta
- property link¶
- links = [<nipy.algorithms.statistics.models.family.links.Log object>, <nipy.algorithms.statistics.models.family.links.Power object>, <nipy.algorithms.statistics.models.family.links.Power object>]¶
- predict(mu)¶
Linear predictors based on given mu values.
- INPUTS:
mu – mean parameter of one-parameter exponential family
- OUTPUTS: eta
- eta – link(mu), linear predictors, based on
mean parameters mu
- tol = 1e-05¶
- valid = [0, inf]¶
- variance = <nipy.algorithms.statistics.models.family.varfuncs.Power object>¶
- weights(mu)¶
Weights for IRLS step.
w = 1 / (link’(mu)**2 * variance(mu))
- INPUTS:
mu – mean parameter in exponential family
- OUTPUTS:
w – weights used in WLS step of GLM/GAM fit